Federated Computer Vision Application
Last updated on
Mar 9, 2022
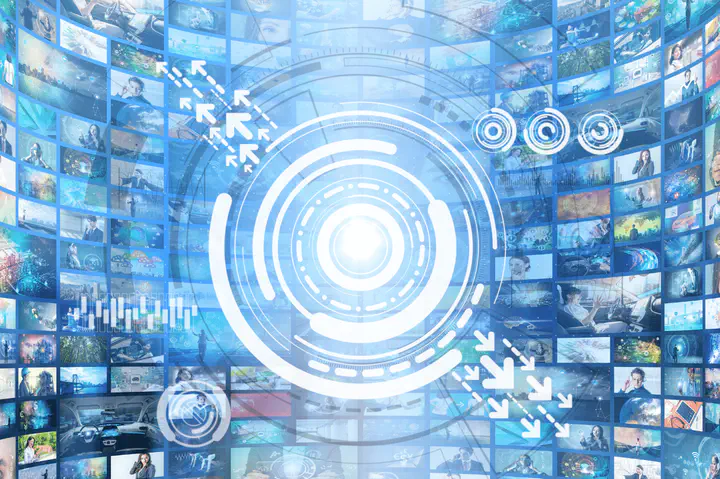
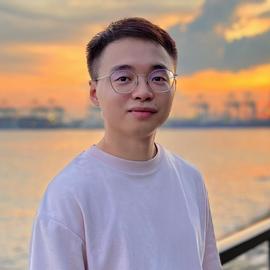
Weiming Zhuang
Senior Research Scientist
My current research interests include multimodal foundation model, federated learning, and computer vision applications.
Publications
We propose federated unsupervised domain adaptation for face recognition, FedFR. FedFR jointly optimizes clustering-based domain adaptation and federated learning to elevate performance on the target domain. Specifically, for unlabeled data in the target domain, we enhance a clustering algorithm with distance constrain to improve the quality of predicted pseudo labels. Besides, we propose a new domain constraint loss (DCL) to regularize source domain training in federated learning.
Weiming Zhuang,
Xin Gan,
Xuesen Zhang,
Yonggang Wen,
Shuai Zhang,
Shuai Yi
We construct a new benchmark to investigate the performance of FedReID, which contains nine datasets with different volumes sourced from different domains to simulate the heterogeneous situation in reality. The benchmark analysis reveals the bottlenecks of FedReID under the real-world scenario, including poor performance of large datasets caused by unbalanced weights in model aggregation and challenges in convergence. To address these issues, we propose two optimization methods: 1) To address the unbalanced weight problem, we propose a new method to dynamically change the weights according to the scale of model changes in clients in each training round; 2) To facilitate convergence, we adopt knowledge distillation to refine the server model with knowledge generated from client models on a public dataset.
Weiming Zhuang,
Yonggang Wen,
Xuesen Zhang,
Xin Gan,
Daiying Yin,
Dongzhan Zhou,
Shuai Zhang,
Shuai Yi